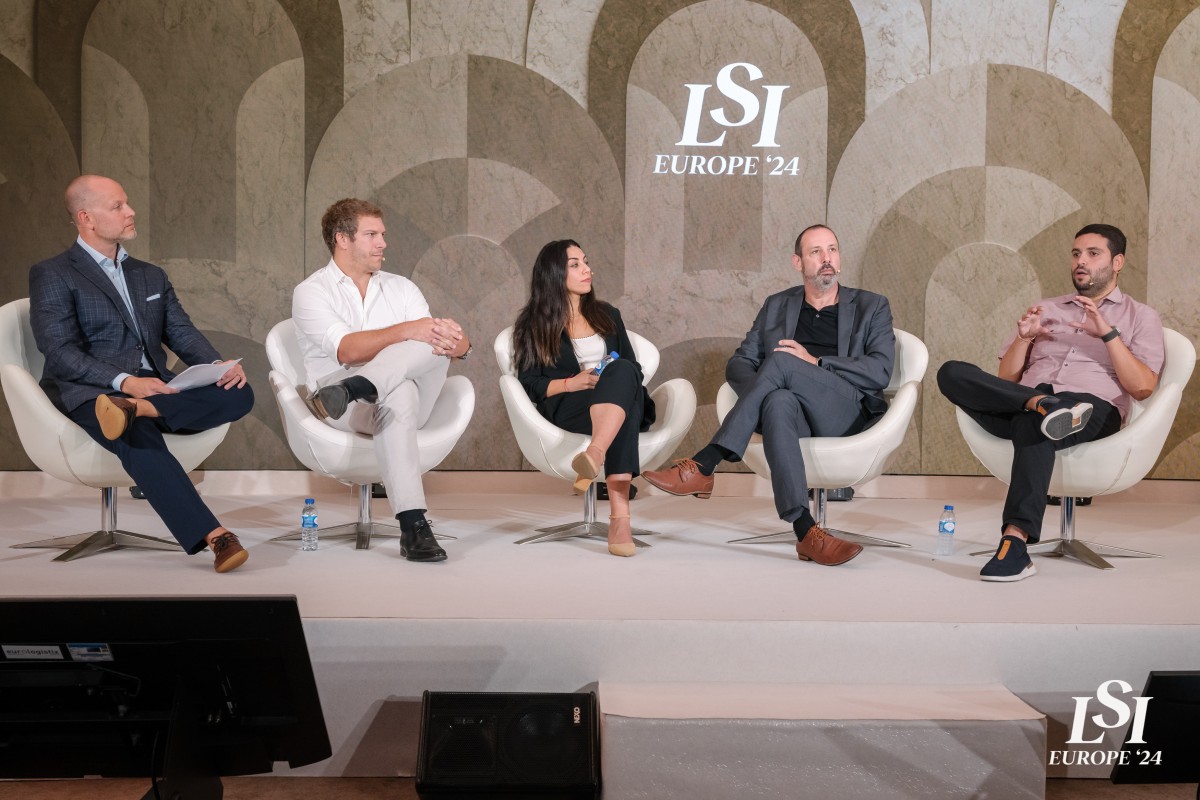
The medtech industry is brimming with AI startups promising to revolutionize healthcare—but how many of those innovations are truly transformative? A panel at LSI Europe ’24 took a clear-eyed look at what it takes to move from promise to impact. The discussion, moderated by Andrew Pieprzyk of Hologic, featured Pau Rodriguez (Methinks AI), Dror Zur (Magentiq Eye), David Zakariaie (Senseye), and Gokce Gun (We Venture Capital), each bringing a distinct vantage point to the table. Together, they offered a grounded yet forward-looking perspective on how artificial intelligence is reshaping diagnostic workflows across stroke, colorectal cancer, mental health, and beyond.
AI in Diagnostics: Trust, Transparency, and Training Data
If AI in diagnostics is to succeed, transparency must be baked into its foundation. Zur, CEO of Magentiq Eye, emphasized that the quality and characteristics of a dataset determine the strength of an AI tool. “It’s important to show what type of data the AI model has been trained and tested on,” he said. “If there are limitations, present them.”
He added that deploying AI without communicating its capabilities and caveats can lead to misdiagnosis—particularly when data is applied outside of its original context. For Rodriguez, building transparency into deployment means confronting tough decisions around scalability and clinical applicability. “We want to solve problems with the best AI possible,” he said, “but then let’s partner. Let’s not reinvent the wheel.”
For Zakariaie, whose company Senseye is developing AI tools for behavioral health, the key is intentional dataset design. “We know the demographics affected by a condition. It’s not rocket science,” he said. “You just need to be intentional about collecting diverse data—and that includes reaching underdiagnosed populations.” In his work with PTSD and anxiety, he noted the FDA’s increasing focus on inclusivity as both a recommendation and, in practice, a requirement.
Post-Market Learning and Real-World Use
Gun, representing We Venture Capital, highlighted the importance of post-market validation. “You can define a representative dataset, but it’s never representative enough,” she said. “Post-market follow-up helps you adapt as your technology evolves in the real world.”
Zur echoed that view, noting that although AI models could theoretically learn online from new data, most systems are designed to update offline. “We supervise the data that we collect, then we train the AI model offline, and then we release it in a very controlled way,” he said, likening the process to releasing new software versions. This ensures that companies maintain responsibility and regulatory compliance, even as models improve.
Integration and Infrastructure
Many of the panelists stressed that the potential of AI technologies in healthcare hinges not just on performance but on integration. Pieprzyk of Hologic noted the complexity of inserting a discrete AI product into a broader care delivery system: “You might have a great model, but how do you work through the nuances of implementation and revenue generation?”
Rodriguez, tackling stroke care, described a solution that embraces rather than replaces existing systems. “We integrated our CT viewer into Microsoft Teams,” he explained. “Why build another communication system when we can scale through one that’s already in 100,000 hospitals?” The goal, he said, is to stay laser-focused on AI’s value while leveraging existing platforms to deliver it.
In mental health, where there are no gold-standard diagnostics, Zakariaie took a similar approach. “We built our product as a software development kit, a plugin for telehealth platforms,” he said. By embedding Senseye’s diagnostics into services like Talkspace and BetterHelp, the team sidesteps the challenges of fragmented primary care and reaches patients where they’re already receiving care.
Business Models That Support Adoption
For companies at the interface of innovation and implementation, the business model is as critical as the product itself. “It’s important to think from the beginning about integration into the clinical flow,” said Zur. “You don’t want to change how doctors work—you want to support them.”
Zur and Zakariaie both highlighted the benefits of leasing or subscription-based models that allow hospitals to try AI tools without significant upfront investment. This flexibility, they noted, encourages the adoption of AI in healthcare while providing recurring revenue to support ongoing development and support.
Customer Success Before Scaling
Before AI companies can scale, they need to prove their systems work in real-world settings—and build deep relationships with early users. Rodriguez explained Methinks AI’s approach: “We hired five new people focused specifically on customer success. It’s more important than sales.” For him, the goal is to deeply understand how physicians use the product, fix what needs fixing, and only then expand.
Zakariaie agreed: “Get feedback from your first customers. Make the product easy to use, then push it to a scale-up.” Gun noted that finding people with the right blend of clinical, technical, and business expertise for customer success roles is a challenge—but a necessary one. “You need someone who can understand everything to a certain level because it’s too complex otherwise.”
Staying Grounded in the Medical Need
As companies scale and strategic investors get involved, there’s always the temptation to chase platforms or revenue streams at the expense of core impact. Gun urged companies to stay rooted in the problem they set out to solve. “It’s not about building AI for the sake of AI,” she said. “You need to look at the medical need—and pursue that.”
Pieprzyk echoed this sentiment: “Only 30% of the global population has access to the care we take for granted. AI gives us the opportunity to reach the rest—to deliver affordable, scalable solutions where none currently exist.”
Zakariaie closed with a vision for mental health diagnostics that speaks to the broader mission of AI in medicine: “Right now, it’s like diagnosing diabetes without ever measuring blood sugar. We want to put real diagnostic tools in the hands of providers—so they can treat patients based on what’s actually happening.”
Conclusion: Meeting the Moment
As the panelists made clear, AI in diagnostics is no longer a hypothetical. It’s here—and it’s evolving. But delivering on its promise requires more than a great algorithm. It demands clarity around data, humility in deployment, creativity in business models, and relentless focus on solving real medical problems. For those navigating this terrain, the opportunity for transformation is real—but only if they stay grounded in clinical value, practical delivery, and global access.
Enjoyed these insights and want to hear more? Consider attending our next medtech conference in Singapore from June 10–13.